Towards Improved Heart Disease Detection: Evaluating Naïve Bayes and K-Nearest Neighbors in Medical Data Classification
DOI:
https://doi.org/10.70103/galaksi.v1i3.45Kata Kunci:
Classification, Comparison Method, Naïve Bayes, K-Nearst NeighborsAbstrak
The application of machine learning in healthcare is increasingly critical for improving diagnostic accuracy and timely treatment. This study explores the classification of heart disease using Naïve Bayes and K-Nearest Neighbors (KNN), focusing on evaluating their effectiveness through a comparative analysis. The research addresses the challenge of identifying an optimal method for heart disease classification, emphasizing the need for reliable algorithms. Using a dataset from Kaggle with detailed preprocessing, we implement Naïve Bayes and KNN to assess classification performance. The study introduces a comparative perspective on classification accuracy, precision, recall, and F1-score, revealing the strengths and limitations of each method. The results highlight the superior performance of Naïve Bayes with an accuracy of 88%, offering novel insights for data-driven healthcare decisions.
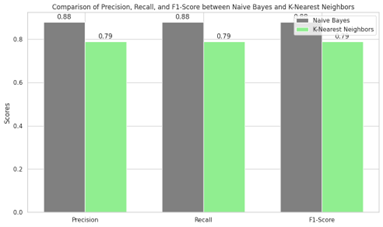