Advanced Long Short-Term Memory (LSTM) Models for Forecasting Indonesian Stock Prices
DOI:
https://doi.org/10.70103/galaksi.v1i3.42Keywords:
Indonesian Stock Market, Long Short-Term Memory (LSTM), Predictive Analytics, Stock Price Forecasting, Time Series AnalysisAbstract
The Indonesian stock market is a key indicator of national economic dynamics. Blue-chip stocks, including Bank Central Asia (BBCA), Bank Rakyat Indonesia (BBRI), and Bank Mandiri (BMRI), hold significant influence due to their liquidity and impact on the market index. However, their price volatility, driven by global economic conditions, monetary policies, and market sentiment, poses challenges for accurate forecasting. This study employs the Long Short-Term Memory (LSTM) model to address these challenges. LSTM, a deep learning technique, effectively handles time series data by capturing long-term dependencies and complex price patterns. Using historical stock data from 2019 to 2024, the model was trained and optimized. Evaluation metrics, including Mean Absolute Percentage Error (MAPE) and Root Mean Squared Error (RMSE), were used to assess performance. BBCA stocks achieved the best results, with a MAPE of 0.0099 and RMSE of 128.02.The findings demonstrate LSTM's robustness in forecasting stock price trends, providing investors with valuable tools for informed decision-making. This research advances predictive analytics in financial markets, particularly in emerging economies like Indonesia, and highlights LSTM’s potential to improve accuracy in volatile environments.
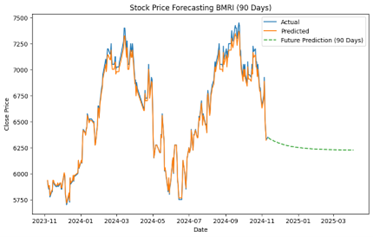