Implementation of Recurrent Neural Network Gated Recurrent Unit (GRU) Model for Predicting Top-Tier Bitcoin
DOI:
https://doi.org/10.70103/galaksi.v1i3.41Keywords:
Cryptocurrency, Forecasting, GRU Method, InvestmentAbstract
Cryptocurrency investments are becoming increasingly popular due to their potential as digital assets, though high price volatility poses significant challenges for investment decision-making. This study employs the Gated Recurrent Unit (GRU) model to forecast the closing prices of five prominent cryptocurrencies: Bitcoin (BTC), Ethereum (ETH), Ripple (XRP), Binance Coin (BNB), and Dogecoin (DOGE), using historical data from Yahoo Finance spanning 2019 to 2024. The model's performance was assessed using evaluation metrics, including Mean Absolute Percentage Error (MAPE), Root Mean Squared Error (RMSE), Mean Absolute Error (MAE), and R-squared (R²). The results demonstrate that BNB achieved the best performance, with a MAPE of 2.38% and RMSE of 17.03, followed by ETH and XRP, which recorded MAPEs of 2.51% and 2.64%, respectively. BTC exhibited the highest RMSE at 2280.73, highlighting its significant price volatility, while DOGE had the lowest RMSE at 0.01, despite recording the highest MAPE at 4.11%. Forecasts for the next six periods indicate that BTC and ETH are likely to experience gradual price increases, XRP and BNB are expected to stabilize, and DOGE will remain relatively stable with low volatility. The study concludes that the GRU model is effective for cryptocurrency price forecasting, but integrating it with fundamental and technical analysis could further enhance accuracy and support more informed investment decisions.
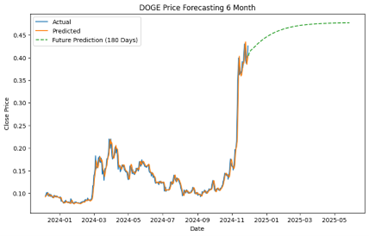